Artificial intelligence (AI) has the potential to alter the landscape of healthcare delivery as we know it. Healthcare leaders must not only understand AI, but also embrace it for their personal leadership growth and to guide their organizations in adapting to the evolving environment. Multiple incentives are driving the healthcare industry to use AI in dealing with financial pressures, clinician shortages, and the efficient mining of vast electronic data.(1)
It is essential to understand AI-related definitions to use this area of computer science better. AI is “the theory and development of computer systems capable of performing tasks that typically require human intelligence, such as visual perception, speech recognition, decision making, and language translation.”(2)
The two subsets of AI are natural language processing and machine learning (ML).(3) Natural language processing converts spoken language into machine-readable data.(4) ML is a subset of AI that allows computers to learn from existing data and improve their performance for a given task over time.(5) It focuses on developing algorithms and models that enable computers to learn and make predictions or decisions without being specifically programmed. ML is self-adaptive as it collects continuous and extensive data, thus further enhancing its analytical capabilities.(6)
Deep learning (DL) is a subset of machine learning that uses neural networks similar to the brain to adapt with little to no human intervention. It compares previous features through input and output layers without human help.(7) In medical settings, ML and DL have the potential to diagnose certain diseases without the help of humans. If programmed correctly, as more data is collected in its database, DL can progressively become quicker and more accurate at detecting certain features of diseases. DL may ultimately support diagnosis and improve efficiency, leading to enhanced patient care. (Figure 1).
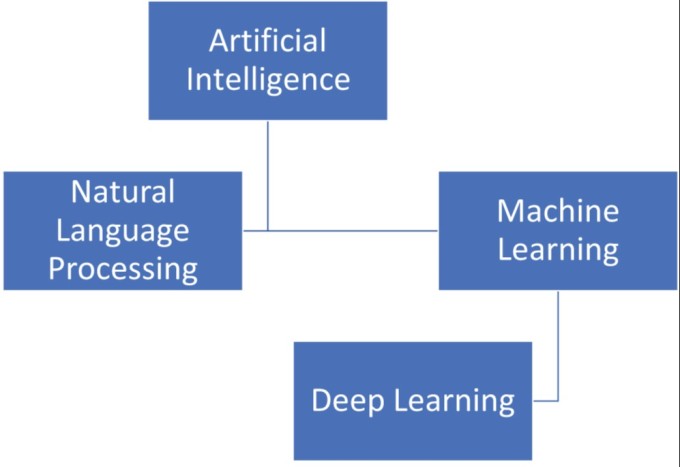
Figure 1. Subsets of AI
IMPACT OF AI ON HEALTHCARE
AI is revolutionizing healthcare in the areas of improved diagnostic algorithms, clinical operations, and treatment options through personalized medicine (Figure 2). AI can potentially allow physicians to function at the top of their clinical skills rather than focusing on clerical work, which has contributed to burnout. AI can reduce repetitive tasks and documentation burdens as it cues up relevant data in anticipation of physician-patient interactions.
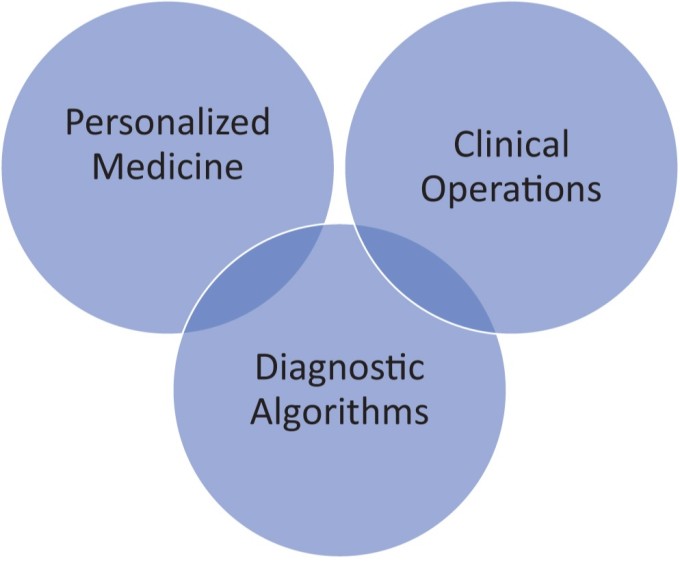
Figure 2. Healthcare Applications of AI
AI can also help physicians with nudges and alerts at the “point of care” to ensure that patient safety and quality care remain at the forefront of every interaction. AI may prioritize and prompt physicians to ensure their compliance with evidence-based medical practices, and it may reinforce best practices by identifying physicians who are complying with them. By reducing administrative burdens, AI may ultimately help reduce the burnout that has contributed to physicians and nurses leaving the field of medicine.
Diagnostic Algorithms
AI is already being used to optimize pathology, gastroenterology, dermatology, and radiology algorithms to improve diagnostic accuracy.
In pathology, AI has shown superior accuracy and efficiency compared to the human eye for pathological interpretation of specimens.(8) The practical benefits of AI integration include widely accessible data, higher accuracy in diagnostics, instantaneous imaging of histology slides, and streamlined pathologist workflow. As technology improves and stabilizes, self-diagnosis with the help of AI may be possible, and early disease detection has potential value to patients with limited access to advanced medical care.
AI software with deep learning-based algorithms has been used to diagnose upper gastrointestinal neoplasia. In one large study, endoscopy data from upper GI biopsy patients were submitted to the deep learning AI program to detect neoplasia. The deep learning program generally demonstrated more accurate and quicker detection of upper GI neoplasia than did human endoscopists. These results predict AI’s critical future role in the diagnosis of mucosal-based diseases. Therefore, AI can be a good “proofreading” tool for individual physicians through expertly coded algorithms.(9)
In dermatology, AI distinguished images of melanomas and nevi that human experts had previously classified. Histopathology images submitted to the AI network for pattern recognition revealed discordance of only 19% among the AI-assisted histopathologists, compared to 25–26% among non-AI-assisted histopathologists. This research further cements the potential of AI in medical settings.(10)
Radiology has been more receptive than other medical specialties to using AI. Van Leeuwen, et al., described several clinical objectives AI can support in radiology.(11) AI can create a more efficient workflow, shorten the reading time, help reduce the dose of contrast agents, optimize earlier disease detection, improve diagnostic accuracy, and provide more personalized diagnostics.
AI’s identification of possible abnormalities in high-risk patients directs the attention of interpreting radiologists.(11) Benefits transcend across radiologists of varying experience levels and, therefore, could lead to more accurate readings with less variation among radiologists.
Personalized Medicine
Personalized or precision medicine refers to medical treatments or predictions focused on a particular patient; AI’s current and future treatment applications relate to personalized medicine. AI is specifically applied to genomic data analysis, clinical decision support systems, drug discovery, remote patient monitoring, and customized rehabilitation and therapy. AI analyzes genomic data to identify genetic markers, aiding in the understanding of diseases and guiding personalized treatment plans.(12)
In clinical decision support systems (CDSS), AI-powered CDSS offers healthcare providers evidence-based recommendations for treatment decisions. In drug discovery, AI expedites drug discovery by analyzing biological data and predicting potential drug candidates.(13)
To keep healthcare costs down, there is a trend toward homecare through remote patient monitoring; AI enables continuous patient monitoring, providing real-time data for chronic disease management and early intervention.(14) In personalized rehabilitation and therapy, AI tailors rehabilitation plans and adapts therapy regimens based on individual patient progress.(15)
Clinical Operations
AI can positively impact clinical operations, as it lessens administrative burdens on the patient-interactive clinical areas and back-office functions. Potentially, AI can improve efficiency, patient safety, and caregivers’ and patients’ experience, all of which are common national metrics.(16,17)
In the clinic, natural language processing can document bedside conversations and physical exam findings, thus creating a complete office note for the physician while enhancing decision support and personalized care plans.(16) AI can improve medication compliance, notify caregivers of potential clinical adverse events, and customize treatment plans based on comprehensive data analysis.(17)
A recent AI study compared physician and AI chatbot responses to patient questions to compare the quality and empathy of the chatbot response to physicians’ written responses. Of note, the chatbot responses were preferred over physician responses and rated significantly higher for quality and empathy.(18) These results suggest that artificial intelligence assistants may aid in drafting responses to patient questions.
AI has great potential to assist with back-office patient functions like patient scheduling, triaging, coding, and billing. The result is a more efficient, cost-effective, and patient-centered healthcare system.
AI CHALLENGES
The healthcare industry must cope with significant challenges related to AI, including data bias, data accuracy, costs, and liability and regulatory concerns (Figure 3).
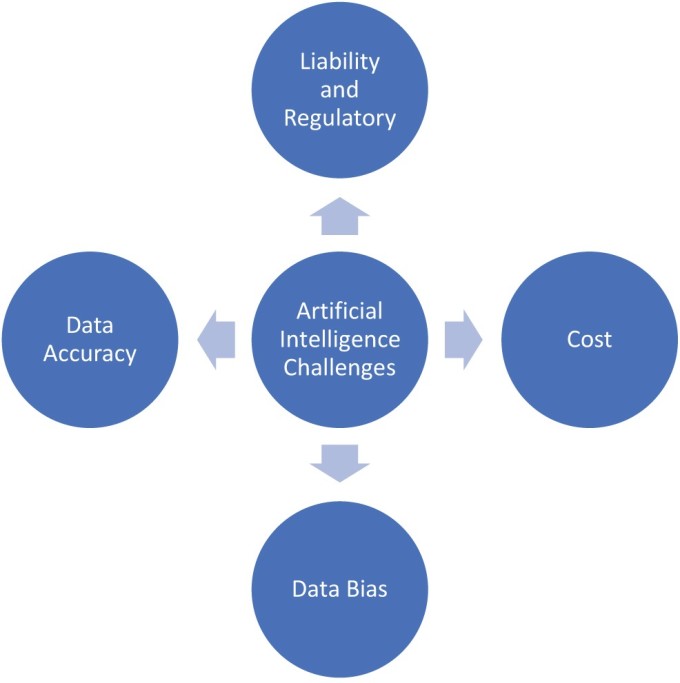
Figure 3. AI Challenges
Data Bias
Data bias can occur when physicians are given incorrect medical information based on biases found in current data related to long-standing historical inequities in healthcare. Many minorities have not had access to healthcare and, as a result, suffer from adverse health outcomes affecting morbidity and mortality rates. Minorities are often underrepresented in training data, and their social determinants of health are not often documented (such as race, age, socioeconomic status, language, education status, geography, disability, sexual orientation, and gender) even though they impact health outcomes.
These concerns may perpetuate bias if future AI algorithms are based on incomplete training data. A solid commitment to diversity, equity, and inclusion is essential when working with AI to prevent the perpetuation of biases in current algorithms.(19)
On the positive side, AI can help reduce health disparities by identifying social determinants of health and using standardized clinical care algorithms to allocate resources fairly. AI can also identify contributors to the disparities and create standard work to ensure resources are distributed according to fair algorithms rather than considering implicit or explicit bias.(20)
Data Accuracy
Artificial intelligence models can lead to “hallucinations” whereby outputs from these models do not make sense or are inaccurate. Hallucinations can result from contradictory information in the training data or out-of-date information in AI models. This can have a profound impact on outcomes; an AI hallucination might incorrectly identify a benign colon polyp as malignant, leading to unnecessary medical interventions.
Some of these concerns related to data accuracy may be mitigated by applying high-quality, vetted training data, deciding on guardrails for the output, and ensuring human validation and verification of outputs are derived from a reliable input source.(21)
Costs
Integrating AI into healthcare generates short-term and long-term costs. The short-term costs are associated with the initial asset investments and staff education. In the long term, maintenance costs must be balanced against long-term cost reduction by streamlining workflows, reducing administrative tasks, and limiting adverse events.(22)
AI may allow the healthcare system to adopt a more upstream approach with a focus on preventative aspects of population healthcare before a disease becomes full-blown. This can be realized by identifying and addressing the risk factors and social determinants of health and through remote patient monitoring, all of which may reduce adverse healthcare events, emergency room visits, office visits, and hospital readmissions. AI may also improve diagnostic accuracy, thereby reducing unnecessary referrals, duplicative tests, and incorrect treatments.
Liability and Regulatory Concerns
The evolving nature of AI in medicine poses challenges for regulatory frameworks and demonstrates the need for specific regulations tailored to AI applications in healthcare. More specifically, these cautions apply to the complex legal concerns of liability and accountability in AI-related errors or adverse outcomes. Legal frameworks may be established to address issues of responsibility.
Handling sensitive patient data with the aid of AI raises concerns about data privacy and security. In the United States, health data is protected as Protected Health Information (PHI) under the Health Insurance Portability and Accountability Act (HIPAA), which governs health data privacy under federal law.(23) Any artificial intelligence system implemented within healthcare must undergo a rigorous examination under the legal lens to ensure that the established standard of data privacy is met. Legal compliance with regulations like HIPAA is crucial.
As a regulatory agency, the FDA oversees medical devices, including AI-based devices. Ensuring appropriate evaluation criteria for safety and efficacy, including provisions for post-market surveillance, is a concern.
Ongoing real-world monitoring for the safety and effectiveness of AI systems for post-market approval is necessary. AI algorithmic errors resulting in medical malpractice are a concern. AI systems can make errors, and determining the responsibility for these errors in a medical context is a consideration in medical malpractice cases.
Finally, providing informed consent and ensuring that patients are aware of the use of AI in their healthcare is conducive to avoiding legal issues.
STRATEGIC IMPLEMENTATION OF AI
A strategic approach is required to integrate AI into a healthcare system (Figure 4). The healthcare leader is responsible for aligning AI with the organization’s overall strategy and not vice versa. A comprehensive needs assessment that includes feedback from the various stakeholders within the organization is necessary.(24)
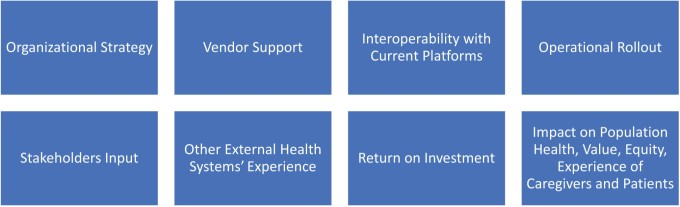
Figure 4. Factors When Considering a New AI Technology
When deciding which new technology to implement, one should do due diligence and, if possible, evaluate other external organizations’ experiences, vendor maintenance support after implementation, infrastructure compatibility, data privacy, cybersecurity, adherence to HIPAA standards, the return on investment and interoperability within the existing electronic health records as well as the impact on population health, value, equity, and the experience of caregivers and patients.(13)
Implementing AI in a specific healthcare area requires validating outcomes, providing staff training, and ongoing monitoring and feedback to meet the objectives. Finally, the framework should be patient-centric to achieve healthcare’s quintuple aim: improving population health, reducing costs, improving the experience for patients and providers, and advancing health equity.(25)
CONCLUSION
The integration of AI across various industries has sparked a revolutionary transformation in how businesses operate, ushering in an era of heightened efficiency. In the manufacturing industry, companies have seamlessly integrated AI machine learning into their work to allow real-time product quality prediction while minimizing equipment downtime through proactive maintenance based on AI recommendations.(26)
In the financial sector, AI has automated financial planning and optimized the budgeting processes. In human resources, AI tools have guided strategic decisions in employee hiring based on potential performance in the company’s unique environment.(27,28) Finally, in the transportation industry, the GPS system is a testament to the power of AI in predicting accurate arrival times in varying traffic conditions.(29)
AI has the potential to positively impact the lives of the healthcare workforce by enhancing efficiency, decision-making, and overall patient care. While AI is changing how we approach healthcare, its impact on healthcare jobs is not straightforward. In the future, AI can automate routine and repetitive tasks to eliminate some administrative positions. Although AI is unlikely to replace physicians entirely, it will make the practice of medicine more efficient.(14) The ultimate legal responsibility for medical decisions lies with physicians who must continue to be involved in healthcare pathways on which AI can be viewed as an assistant or a valuable adjunct, augmenting a physician’s capabilities.(14,30)
While AI may not replace physicians, it behooves physicians to stay up-to-date with AI and integrate it into their practice to ensure they are at the forefront of clinical care. AI will undoubtedly transform the healthcare industry, and leaders must understand not only AI, but also the risks associated with implementing AI in an attempt to ensure their organization remains relevant, competitive, agile, and up-to-date in this fast-paced, changing landscape.
Keith Horvath, MD, from the Association of American Medical Colleges, summed it up best when he said, “AI is not going to replace leaders, but leaders who use AI are going to replace leaders who don’t.”
References
Stanfill MH, Marc DT. Health Information Management: Implications of Artificial Intelligence on Healthcare Data and Information Management. Yearb Med Inform. 2019;28(1):56–64. https://doi.org/10.1055/s-0039-1677913
Russell SJ, Norvig P. Artificial Intelligence: A Modern Approach. Upper Saddle River, NJ: Pearson; 2016.
Goodfellow I, Bengio Y, Courville A. Deep Learning (Vol. 1). Cambridge, MA: MIT Press; 2016.
Jurafsky D, Martin JH. Speech and Language Processing. Upper Saddle River, NJ: Pearson; 2020.
Ahmad Z, Rahim S, Zubair M, Abdul-Ghafar J. Artificial Intelligence (AI) in Medicine, Current Applications and Future Role with Special Emphasis on Its Potential and Promise in Pathology: Present and Future Impact, Obstacles Including Costs and Acceptance Among Pathologists, Practical and Philosophical Considerations. A Comprehensive Review. Diagn Pathol. 2021 Mar 17;16(1):24. https://doi.org/10.1186/s13000-021-01085-4 . PMID: 33731170; PMCID: PMC7971952.
Murphy A, Liszewski B. Artificial Intelligence and the Medical Radiation Profession: How Our Advocacy Must Inform Future Practice. J Med Imaging Radiat Sci. 2019;50(4 Suppl 2): S15–S19. https://doi.org/10.1016/j.jmir.2019.09.001
Hosny A, Parmar C, Quackenbush J, Schwartz LH, Aerts HJWL. Artificial Intelligence in Radiology. Nat Rev Cancer. 2018;18(8):500–510. https://doi.org/10.1038/s41568-018-0016-5
Baxi V, Edwards R, Montalto M, Saha S. Digital Pathology and Artificial Intelligence in Translational Medicine and Clinical Practice. Mod Pathol. 2022;35(1):23–32. https://doi.org/10.1038/s41379-021-00919-2
Sharma P, Hassan C. Artificial Intelligence and Deep Learning for Upper Gastrointestinal Neoplasia. Gastroenterology. 2022;162(4):1056–1066. https://doi.org/10.1053/j.gastro.2021.11.040
Hekler A, Utikal JS, Enk AH, et al. Superior Skin Cancer Classification by the Combination of Human and Artificial Intelligence. Eur J Cancer. 2019;120:114–121. https://doi.org/10.1016/j.ejca.2019.07.019
Van Leeuwen KG, DeRooij M, Schalekamp S, et al. How Does Artificial Intelligence in Radiology Improve Efficiency and Health Outcomes? Pediatr Radiol. 2002;52:2087–2093. https://doi.org/10.1007/s00247-021-05114-8
Min S, Lee B, Yoon S. Deep Learning in Bioinformatics. Brief Bioinform. 2017;18(5):851–869. https://doi.org/10.1093/bib/bbw068
Chen IY, Joshi S, Gharakheili HH. AI in Healthcare: A Review. Artif Intell Med. 2021;111:101995.
Topol EJ. High-performance Medicine: The Convergence of Human and Artificial Intelligence. Nat Med. 2019;25(1):44–56. https://doi.org/10.1038/s41591-018-0300-7
Varghese J, Bajpai A, Johnson WJ. Adaptive Learning for Personalized Rehabilitation of the Elbow Using a Haptic Bracelet. In: Proceedings of the 9th International Conference on Agents and Artificial Intelligence (ICAART 2017). 1. 469–476.
Dernoncourt F, Lee JY, Uzuner Ö, Szolovits P. De-identification of Patient Notes with Recurrent Neural Networks. J Am Med Inform Assoc. 2017;24(3):596–606. https://doi.org/10.1093/jamia/ocw156
Miotto R, Li L, Kidd BA, Dudley JT. Deep Patient: An Unsupervised Representation To Predict the Future of Patients from the Electronic Health Records. Sci Rep. 2017;6(1):1–10. https://doi.org/10.1038/srep26094
Ayers JW, Poliak A, Dredze M, et al. Comparing Physician and Artificial Intelligence Chatbot Responses to Patient Questions Posted to a Public Social Media Forum. JAMA Intern Med. 2023;183(6):589–596. https://doi.org/10.1001/jamainternmed.2023.1838
Rudin C, Ustun B. Opportunities and Challenges in Machine Learning–Driven Personalized Medicine. Sci Transl Med. 2019; 11(477).
Obermeyer Z, Powers B, Vogeli C, Mullainathan S. Dissecting Racial Bias in an Algorithm Used to Manage the Health of Populations. Science. 2019;366(6464):447–453. https://doi.org/10.1126/science.aax2342
Hatem R, Simmons B, Thornton JE. A Call to Address AI “Hallucinations” and How Healthcare Professionals Can Mitigate Their Risks. Cureus. 2023;15(9):e44720. https://doi.org/10.7759/cureus.44720
Chen H, Engkvist O, Wang Y, Olivecrona M, Blaschke T. The Rise of Deep Learning in Drug Discovery. Drug Discov Today. 2018;23(6):1241–1250. https://doi.org/10.1016/j.drudis.2018.01.039
Marks M, Haupt CE. AI Chatbots, Health Privacy, and Challenges to HIPAA Compliance. JAMA. 2023;330(4):309–310. https://doi.org/10.1001/jama.2023.9458
Kaplan A, Haenlein M. Siri, Siri, in My Hand: Who Is Fairest in the Land? On the Interpretations, Illustrations, and Implications of Artificial Intelligence. Bus Horiz. 2019;62(1):15–25. https://doi.org/10.1016/j.bushor.2018.08.004
Choudhury A, Asan O. Role of Artificial Intelligence in Patient Safety Outcomes: Systematic Literature Review. JMIR Med Inform. 2020;8(7):e18599. https://doi.org/10.2196/18599
Woods B, Turekian V, Jeong T, Gronvall GK, et al. Building a Smart Partnership for the Fourth Industrial Revolution. Adding Value with Artificial Intelligence. Washington, DC: Atlantic Council; 2018: 15–21.
Agrawal A, Gans J, Goldfarb A. Artificial Intelligence: The Ambiguous Labor Market Impact of Automating Prediction. J Econ Persp. 2019;33(2):31–50. https://doi.org/10.1257/jep.33.2.31
Tyson LD, Zysman J. Automation, AI & Work. Daedalus. 2022;151(2): 256–271. https://doi.org/10.1162/daed_a_01914
Heller CH. Near-Term Applications of Artificial Intelligence: Implementation Opportunities from Modern Business Practices. Naval War College Review. 2019;72(4):73–100.
Obermeyer Z, Emanuel EJ. Predicting the Future—Big Data, Machine Learning, and Clinical Medicine. N Engl J Med. 2016;375(13):1216–1219. https://doi.org/10.1056/NEJMp1606181